- CSCD核心中文核心科技核心
- RCCSE(A+)公路运输高质量期刊T1
- Ei CompendexScopusWJCI
- EBSCOPж(AJ)JST
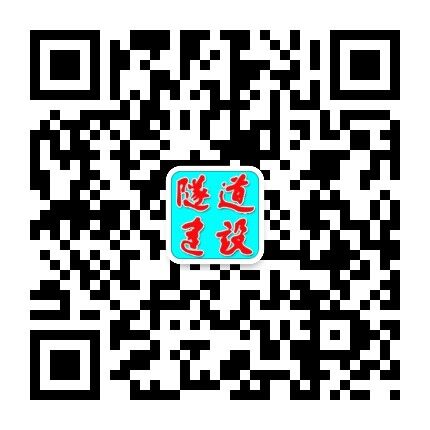
隧道建设(中英文) ›› 2025, Vol. 45 ›› Issue (3): 499-510.DOI: 10.3973/j.issn.2096-4498.2025.03.005
洪成雨1, 2, 黎宏1, 2,*, 饶伟1, 2, 陈湘生1, 2, 雷振3
HONG Chengyu1, 2, LI Hong1, 2, *, RAO Wei1, 2, CHEN Xiangsheng1, 2, LEI Zhen3
摘要: 为研究地铁地下车站大尺寸装配式构件在拼装过程中的应变特征与裂纹识别问题,以某装配式地铁车站工程为研究背景,针对大尺寸构件拼装过程中的分布式光纤(distributed fiber optic system,DFOS)监测开展研究,提出主成分层次聚类模型(PCA-HC),融合多个应变分布参数,对裂纹区域应变分布进行特征识别,并开展现场底板拼装裂纹验证。研究结果表明: 1)根据DFOS获取的应变特征,拼装顶板时受拉区平均应变增长率为105.42%,受压区平均应变增长率为110.27%。就底板而言,顶板拼装对其变形影响最大。2)DFOS清晰地反映了裂纹应变异常信号,拼装过程中底板与支撑结构接触处出现裂纹,建议增大支座与拼装构件的接触面积,以减小拼装施工对结构应力/应变集中的影响。3)PCA-HC模型相互融合的降维、特征提取和层次化聚类方法有效识别了裂纹潜在特征,实现了大尺寸装配式构件的裂纹自动化识别与分类,为保障地铁车站大尺寸装配式结构安全施工提供了方法。