ISSN 2096-4498
CN 44-1745/U
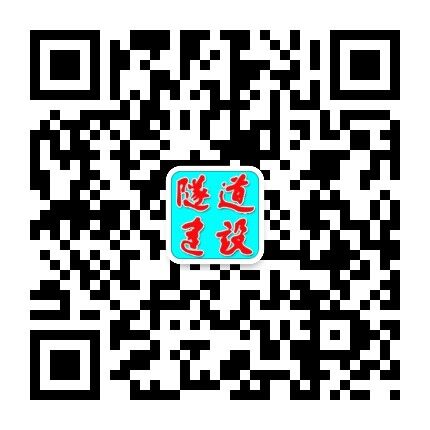
ISSN 2096-4498
CN 44-1745/U
Tunnel Construction ›› 2022, Vol. 42 ›› Issue (1): 33-40.DOI: 10.3973/j.issn.2096-4498.2022.01.005
Previous Articles Next Articles
Rapid Classification Technology of Surrounding Rock of Tunnel Face Based on ThreeDimensional Reconstruction and Unet Neural Networks
LI Chimou1, 2, LYU Ming1, 2, YUAN Qing2, CHEN Yujia3, *, WANG Shuying3
(1. Wenshan Expressway Construction and Development of CCCC Co., Ltd., Wenshan 663099, Yunnan, China; 2. CCCC Second Harbor Engineering Company Ltd., Wuhan 430040, Hubei, China; 3. School of Civil Engineering, Central South University, Changsha 410075, Hunan, China)