ISSN 2096-4498
CN 44-1745/U
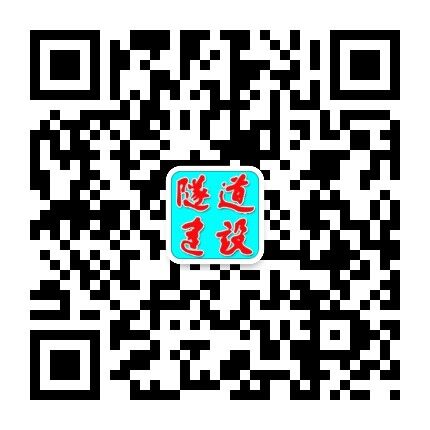
ISSN 2096-4498
CN 44-1745/U
Tunnel Construction ›› 2022, Vol. 42 ›› Issue (8): 1443-1452.DOI: 10.3973/j.issn.2096-4498.2022.08.014
Previous Articles Next Articles
DoubleLayer Quality Evaluation of Surrounding Rock of a Tunnel Based
on Advance Drilling and Optimized Integration Algorithm
LIANG Ming, PENG Hao, XIE Weiwei*,
SONG Guanxian, ZHU Menglong, ZHANG Yafei
(Guangxi Road and Bridge Engineering Group Co., Ltd., Nanning 530000, Guangxi, China)