ISSN 2096-4498
CN 44-1745/U
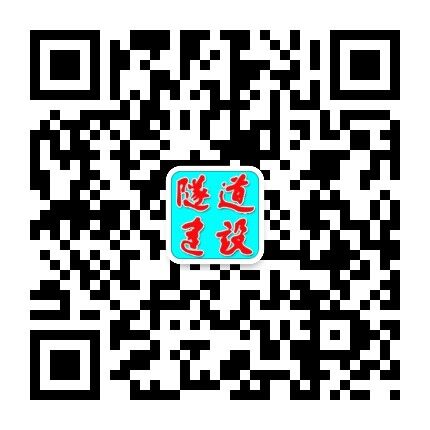
ISSN 2096-4498
CN 44-1745/U
Tunnel Construction ›› 2023, Vol. 43 ›› Issue (8): 1369-1378.DOI: 10.3973/j.issn.2096-4498.2023.08.011
Previous Articles Next Articles
Freezing Characteristics and Freezing Depth Prediction of Tunnels in Cold Regions
MA Chao1, LI Zhonghao1, QIU Junling1, *, LAI Jinxing1, LUO Yanping2,
ZENG Bin2, FENG Zhihua3
(1. School of Highway, Chang′an University, Xi′an 710064, Shaanxi, China; 2. Sichuan Chuanjiao Road & Bridge Co., Ltd., Guanghan 618300, Sichuan, China; 3. Hebei Provincial Communications Planning, Design and Research Institute Co., Ltd., Shijiazhuang 050011, Hebei, China)