ISSN 2096-4498
CN 44-1745/U
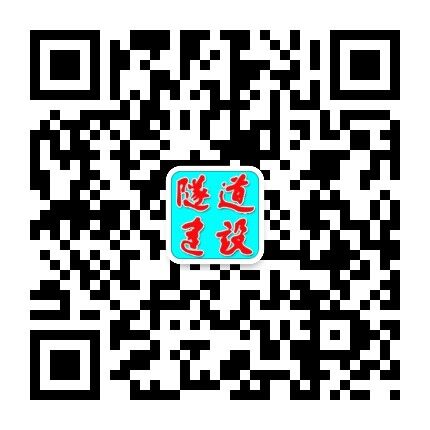
ISSN 2096-4498
CN 44-1745/U
Tunnel Construction ›› 2024, Vol. 44 ›› Issue (5): 1012-1028.DOI: 10.3973/j.issn.2096-4498.2024.05.010
Previous Articles Next Articles
Shapley Additive Explanations Interpretation Model for Penetration Rate Prediction of Tunnel Boring Machines Based on Variational
Mode Decomposition and Weighted Random Forest
ZHANG Jianming1, 2, 3,
SHI Kebin2, 3, *, JIA Yunfu1, REN Zhiqiang4, BAHETEBIEKE Dalayihan4, LIU Zhao
(1. Xinjiang Water Conservancy and Hydropower Survey Design Institute Co., Ltd., Urumqi 830000, Xinjiang, China; 2. College of Water Conservancy and Civil Engineering, Xinjiang Agricultural University, Urumqi 830052, Xinjiang, China; 3. Xinjiang Key Laboratory of Hydraulic Engineering Security and Water Disaster Prevention, Urumqi 830052, Xinjiang, China; 4. Xinjiang Shuifa Construction Group Co., Ltd., Urumqi 830063, Xinjiang, China)