ISSN 2096-4498
CN 44-1745/U
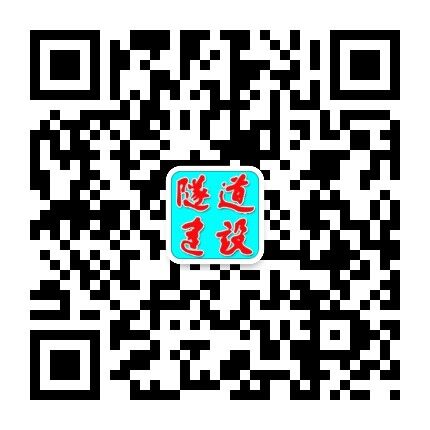
ISSN 2096-4498
CN 44-1745/U
Tunnel Construction ›› 2024, Vol. 44 ›› Issue (S2): 286-299.DOI: 10.3973/j.issn.2096-4498.2024.S2.028
Previous Articles Next Articles
Application of Improved Pelican Optimization Algorithm-Convolutional Neural Networks-Bidirectional Gated Recurrent Unit Model in Predicting Foundation Pit Dewatering
YU Weihe1, HUANG Zhiqiang2, *, WANG Naiyi2, ZHANG Ning2
(1. PetroChina Liaoning Dandong Sales Branch, Dandong 118016, Liaoning, China; 2. School of Architecture and Civil Engineering, Shenyang University of Technology, Shenyang 110870, Liaoning, China)