ISSN 2096-4498
CN 44-1745/U
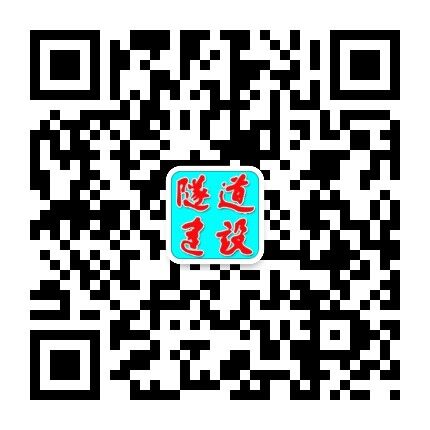
ISSN 2096-4498
CN 44-1745/U
Tunnel Construction ›› 2025, Vol. 45 ›› Issue (3): 579-586.DOI: 10.3973/j.issn.2096-4498.2025.03.013
Previous Articles Next Articles
XU Kunjie1, ZHANG Zhirong1, XIANG Lulu2, 3, *, CHENG Haibing2, 3, TONG Jianjun2, 3, YE Pei2, 3, MIAO Xingwang2, 3