ISSN 2096-4498
CN 44-1745/U
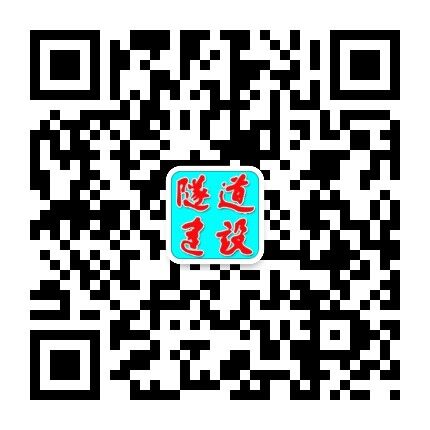
ISSN 2096-4498
CN 44-1745/U
Tunnel Construction ›› 2025, Vol. 45 ›› Issue (4): 677-686.DOI: 10.3973/j.issn.2096-4498.2025.04.002
Previous Articles Next Articles
HONG Kairong1, 2, 3, WANG Kai1, 2, 3, *, LI Zhanguo1, 2, 3, CHEN Ruixiang1, 2, 3, LI Fengyuan1, 2, 3, CHEN Qiao1, 2, 3, QIN Yinping1, 2, 3