ISSN 2096-4498
CN 44-1745/U
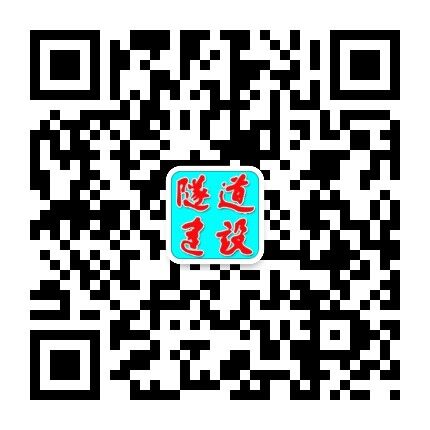
ISSN 2096-4498
CN 44-1745/U
Tunnel Construction ›› 2020, Vol. 40 ›› Issue (S1): 247-253.DOI: 10.3973/j.issn.2096-4498.2020.S1.031
Previous Articles Next Articles
ZHANG Junbo, HE Chuan, YAN Jian*, WU Fangyin, MENG Wei