ISSN 2096-4498
CN 44-1745/U
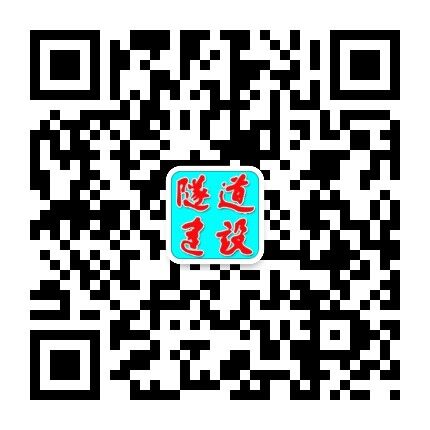
ISSN 2096-4498
CN 44-1745/U
Tunnel Construction ›› 2021, Vol. 41 ›› Issue (S2): 246-254.DOI: 10.3973/j.issn.2096-4498.2021.S2.031
Previous Articles Next Articles
LIU Chunmei1, JIANG Wei1, GONG Yafeng2, *, LIN Siyuan2
(1. China Railway Tunnel Group Road & Bridge Engineering Co., Ltd., Tianjin 300308, China; 2. Transportation College of Jilin University, Changchun 130022, Jilin, China)