ISSN 2096-4498
CN 44-1745/U
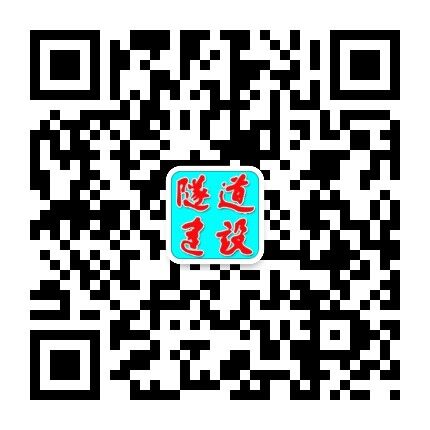
ISSN 2096-4498
CN 44-1745/U
Tunnel Construction ›› 2022, Vol. 42 ›› Issue (11): 1863-1870.DOI: 10.3973/j.issn.2096-4498.2022.11.005
Previous Articles Next Articles
Prediction and Engineering Application of Permeability Coefficients in Improved Muck of Shield Based on Random Forest Algorithm
ZHANG Wentao1, GONG Zhenyu2, LING Fanlin3, *, WANG Shuying3
(1.Kunming Branch of Central Yunnan Provincial Water Diversion Project Construction Administration,Kunming 650051,Yunnan, China;2.Electricity Engineering Co.,Ltd. of CREC No.5 Group,Changsha 410205,Hunan,China;3.School of Civil Engineering, Central South University,Changsha 410075,Hunan,China)