ISSN 2096-4498
CN 44-1745/U
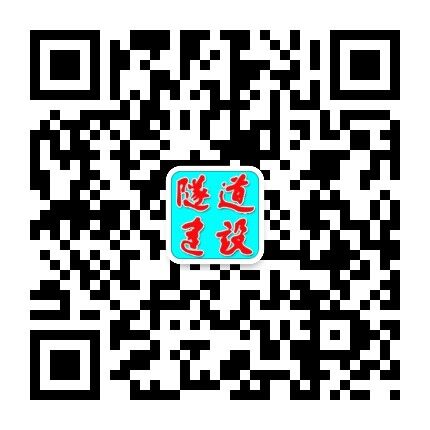
ISSN 2096-4498
CN 44-1745/U
Tunnel Construction ›› 2023, Vol. 43 ›› Issue (3): 514-520.DOI: 10.3973/j.issn.2096-4498.2023.03.016
Previous Articles Next Articles
MA Zhuo1, FANG Zhongqiang2, ZHANG Dan1, *, TU Qiliang2, SHI Bin1, NURLAN Yedili1
(1.School of Earth Science and Engineering,Nanjing University, Nanjing 210023,Jiangsu,China;2.China Design Group Co.,Ltd., Research and Development Center of Transport Industry of Technologies and Equipments for Intelligent Design,Construction and Maintenance of Underwater Tunnel,Ministry of Transport,Nanjing 210014,Jiangsu,China)