ISSN 2096-4498
CN 44-1745/U
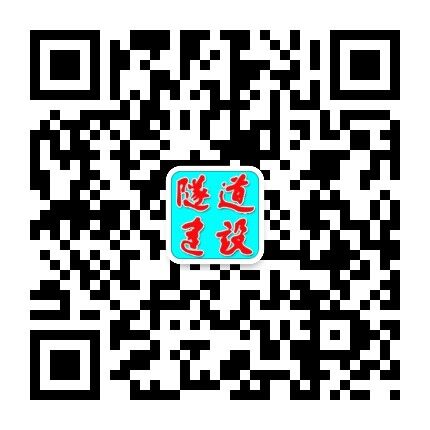
ISSN 2096-4498
CN 44-1745/U
Tunnel Construction ›› 2023, Vol. 43 ›› Issue (9): 1485-1491.DOI: 10.3973/j.issn.2096-4498.2023.09.005
Previous Articles Next Articles
QIAO Jinli1, CHEN Shuai1, CHEN Xiaoqiang2, *, HAO Gangli3, HU Jianbang1, SUN Yongtao1