ISSN 2096-4498
CN 44-1745/U
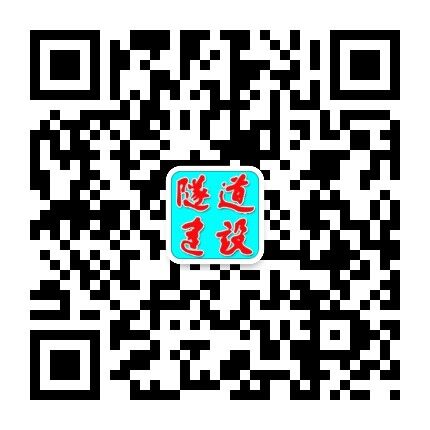
ISSN 2096-4498
CN 44-1745/U
Tunnel Construction ›› 2024, Vol. 44 ›› Issue (9): 1752-1761.DOI: 10.3973/j.issn.2096-4498.2024.09.004
Previous Articles Next Articles
Knowledge-Data-Driven Analysis Method for Safety Status of Immersed Tube Tunnel Joints: A Case Study of Subsea Immersed Tube Tunnel of Hong Kong-Zhuhai-Macao Bridge
DING Hao1, 2, ZHOU Chenyi1, 2, *, GUO Hongyan1, 2, ZHOU Yunteng1, 2
(1. China Merchants Chongqing Communications Technology Research & Design Institute Co., Ltd., Chongqing 400067, China; 2. National Engineering Research Center for Road Tunnel, Chongqing 400067, China)