ISSN 2096-4498
CN 44-1745/U
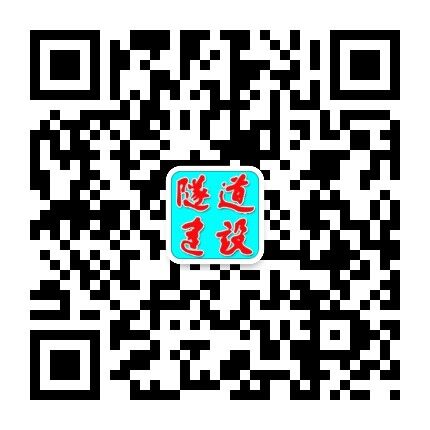
ISSN 2096-4498
CN 44-1745/U
Tunnel Construction ›› 2024, Vol. 44 ›› Issue (8): 1576-1586.DOI: 10.3973/j.issn.2096-4498.2024.08.05
Previous Articles Next Articles
Monitoring Method for Shield Tunneling Operations Based on a Double-Layer Long Short-Term Memory Network
LIU Sijin