ISSN 2096-4498
CN 44-1745/U
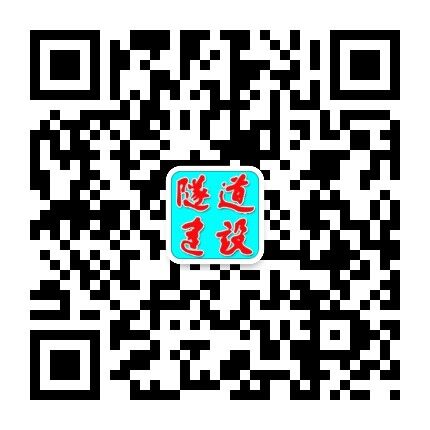
ISSN 2096-4498
CN 44-1745/U
Tunnel Construction ›› 2025, Vol. 45 ›› Issue (2): 221-255.DOI: 10.3973/j.issn.2096-4498.2025.02.001
Previous Articles Next Articles
SONG Zhanping1, 2, 3, YANG Zifan2, ZHANG Yuwei1, 2, *, HUO Runke1, 2