ISSN 2096-4498
CN 44-1745/U
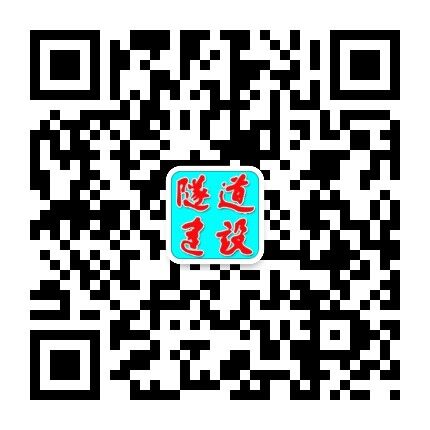
ISSN 2096-4498
CN 44-1745/U
Tunnel Construction ›› 2023, Vol. 43 ›› Issue (12): 1996-2006.DOI: 10.3973/j.issn.2096-4498.2023.12.002
Previous Articles Next Articles
SelfCorrecting Point Cloud for Dump Carriage Based on Deep Convolutional SelfCoding Network
ZHAO Shuanfeng, WANG Shuaijun, LI Yang, WU Yuyao, WANG Mengwei