- CSCD核心中文核心科技核心
- RCCSE(A+)公路运输高质量期刊T1
- Ei CompendexScopusWJCI
- EBSCOPж(AJ)JST
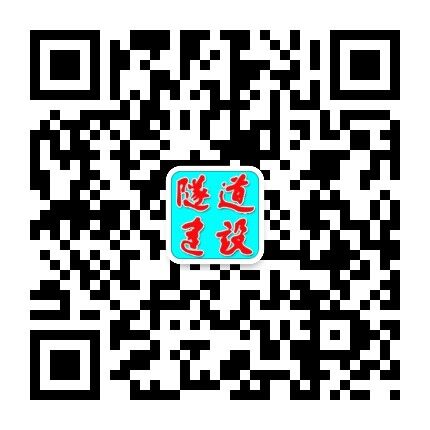
隧道建设(中英文) ›› 2023, Vol. 43 ›› Issue (4): 563-582.DOI: 10.3973/j.issn.2096-4498.2023.04.003
申艳军1, 2, 吕游1, 李曙光3, *, 张津源4, 马文1, 张蕾1, 许振浩4, 黄勇5
SHEN Yanjun1, 2, LYU You1, LI Shuguang3, *, ZHANG Jinyuan4, MA Wen1, ZHANG Lei1, XU Zhenhao4, HUANG Yong5
摘要: 目前,隧道围岩分级方法有评价围岩稳定性分级方法和评价围岩可挖性分级方法2类,分别对应钻爆法施工围岩分级方法和TBM法施工围岩分级方法。据此,围绕现阶段2类方法研究进展予以分别阐述。首先,针对钻爆法施工围岩分级方法,介绍其发展现状和常用分级方法适用条件,并提出3条后续发展建议: 1)重视工程因素对围岩分级劣化的定量表征; 2)积极探索围岩亚级精细化分级方法; 3)推进围岩分级方法集成化体系构建。然后,针对服务于围岩可挖性的TBM法施工围岩分级,梳理基于围岩可挖性及TBM施工适应性的常见围岩分级方法,并着重关注考虑关键地质因素、TBM掘进参数和渣料特征的TBM综合分级方法。其中,可挖性分级建议以TBM净掘进速度、岩体可挖性和现场贯入度指数等参数作为评价指标,而适应性分级则建议依据卡机风险、TBM利用率或施工速度进行评价。最后,介绍“人工智能+”在隧道工程围岩分级方法的应用动态,探索隧道围岩分级集成算法模型、智能化图像处理技术、云计算、智能决策等新方法及新技术在隧道工程围岩分级方法领域的最新应用动态,并提出“人工智能+”在隧道工程围岩分级领域的未来发展方向。