- CSCD核心中文核心科技核心
- RCCSE(A+)公路运输高质量期刊T1
- Ei CompendexScopusWJCI
- EBSCOPж(AJ)JST
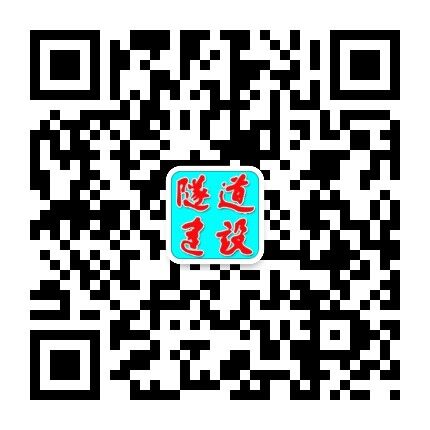
隧道建设(中英文) ›› 2025, Vol. 45 ›› Issue (3): 579-586.DOI: 10.3973/j.issn.2096-4498.2025.03.013
徐昆杰1, 张治荣1, 向露露2, 3, *, 程海兵2, 3, 童建军2, 3, 叶沛2, 3, 苗兴旺2, 3
XU Kunjie1, ZHANG Zhirong1, XIANG Lulu2, 3, *, CHENG Haibing2, 3, TONG Jianjun2, 3, YE Pei2, 3, MIAO Xingwang2, 3
摘要: 为综合利用隧道勘察及施工阶段的地质信息,提升掌子面围岩级别判识的准确性,提出一种基于钻进参数与超前地质预报地震波反射法(TSP)融合的围岩级别智能判识方法。首先,从施工现场收集钻进参数、TSP报告及掌子面地质素描,读取相应的数据与围岩级别信息。其次,为明确钻进参数与地震波反射法的融合方式,设置5种融合工况,并选择4种机器学习分类算法(K近邻、梯度提升树、随机森林、极限树)验证融合数据的可分性。考虑到数据划分方式对模型的拟合能力有影响,通过设置随机数种子对数据集进行500种划分,以使各模型充分拟合。研究结果表明: 采用钻进参数中的进给速度、推进压力、打击压力及回转压力与TSP中纵波速度、横波速度进行融合的工况下,模型分类效果最好,最大准确率为91.3%(采用极限树模型)。最后,使用贝叶斯优化算法对极限树模型的超参数进行优化,通过对比分析,经过优化后的模型在各个围岩级别上的分类性能均有所提升,且总体准确率为93.27%,提升了1.93%。