- CSCD核心中文核心科技核心
- RCCSE(A+)公路运输高质量期刊T1
- Ei CompendexScopusWJCI
- EBSCOPж(AJ)JST
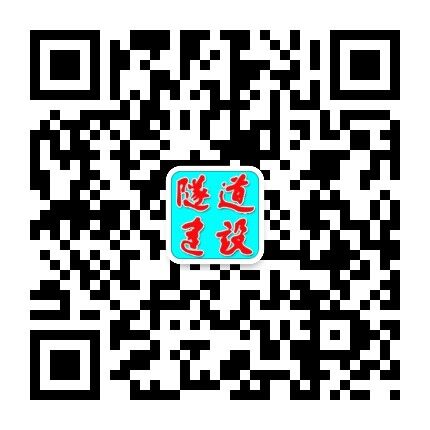
隧道建设(中英文) ›› 2024, Vol. 44 ›› Issue (10): 1971-1981.DOI: 10.3973/j.issn.2096-4498.2024.10.006
傅金阳1, 2, 王浩宇1, 谢纪辰1, 祝志恒1, 张聪3, 伍军4
FU Jinyang1, 2, WANG Haoyu1, XIE Jichen1, ZHU Zhiheng1, ZHANG Cong3, WU Jun4
摘要: 为解决水工隧洞尺寸较小、断面形式多变、洞内环境复杂、无信号覆盖给检测图像快速采集带来的难题,研制出一种无信号覆盖下图像实时传输以及适用于不同断面、尺寸下隧洞全断面采集的水工隧洞检测系统,采集重叠率和精度满足三维重建及对自主研发的衬砌展开影像生成器的要求;针对隧洞表观病害传统算法速度慢、精度低等问题,引入DenseNet主干网络和ECA注意力机制对YOLOv5检测算法进行改进,优化特征提取能力;针对单张图像识别难以反映病害整体分布、全景展开图尺寸过大网络难以捕获有效特征等问题,以全景展开图底层图像为种子点,利用方向搜索结合目标检测算法提出一种“段-环-点”的隧洞常见表观病害快速识别算法。现场应用表明: 1)相比YOLOv7-tiny、YOLOv8、YOLOv9等模型,改进的YOLOv5模型在mAP和F1上均有明显提高,可有效提升复杂背景下病害识别与边界定位精度,显著减少误检和漏检现象; 2)所提技术适用于圆涵、箱涵等不同类型、尺寸的水工隧洞表观图像快速获取与全景识别,能够用于隧洞表观病害的展示和快速定位,可为病害整治措施的及时制定提供依据。