- CSCD核心中文核心科技核心
- RCCSE(A+)公路运输高质量期刊T1
- Ei CompendexScopusWJCI
- EBSCOPж(AJ)JST
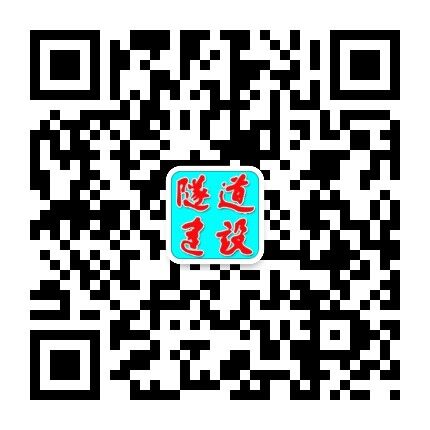
隧道建设(中英文) ›› 2023, Vol. 43 ›› Issue (12): 2007-2017.DOI: 10.3973/j.issn.2096-4498.2023.12.003
张树才1, 仇文革 2, 3, 张齐芳4, 张义江5, 江书华3, 罗杰2, *
(1. 皖赣铁路安徽有限责任公司, 安徽 合肥 230011; 2. 成都天佑智隧科技有限公司, 四川 成都 610031; 3. 西南交通大学 交通隧道工程教育部重点实验室, 四川 成都 610031; 4. 中铁上海设计院集团有限公司, 上海 200070; 5. 中铁十局集团第三建筑有限公司, 安徽 合肥 250101)
Rock Strength Prediction Model Based on Drilling Parameters of Rock Drilling Jumbo
ZHANG Shucai1, QIU Wenge2, 3, ZHANG Qifang4, ZHANG Yijiang5, JIANG Shuhua3, LUO Jie2, *
(1.Anhui-Jiangxi Railway Anhui Co.,Ltd.,Hefei 230011,Anhui,China;2.Chengdu Tianyou Tunnel Key Company Ltd.,Chengdu 610031,Sichuan,China;3.Key Laboratory of Transportation Tunnel Engineering,the Ministry of Education,Southwest Jiaotong University, Chengdu 610031,Sichuan,China;4.China Railway Shanghai Design Institute Group Corporation Limited,Shanghai 200070,China;5.No.3 Construction Company of China Railway No.10 Engineering Group,Hefei 250101,Anhui,China)
摘要: 为及时、准确、全面获取掌子面岩石单轴饱和抗压强度,并为推进隧道智能化建造、实现围岩级别动态变更提供重要依据,依托池黄高铁2标段岭上村隧道工程,获取42组掌子面以及4组岩石相似材料的凿岩台车钻进参数和岩石强度数据。在对凿岩台车的破岩过程进行分析后,选取冲击压力、推进压力、回转压力、钻进速率共4项指标作为预测模型的输入参数,分别建立单因素回归、多元非线性回归和LSSVM算法的岩石强度预测模型。模型预测结果表明: 1)单因素预测模型中采用钻进速率作为输入参数具有最高的预测精度,预测值与实际值的相关系数为0.945 8; 2)采用多因素预测模型后,其预测准确率进一步提高,相关系数达到0.974 5; 3)LSSVM算法模型的预测值与实际值的相关系数为0.983 8,其预测准确率与多元非线性回归相当,均可用于实际工程中的岩石强度预测。