- CSCD核心中文核心科技核心
- RCCSE(A+)公路运输高质量期刊T1
- Ei CompendexScopusWJCI
- EBSCOPж(AJ)JST
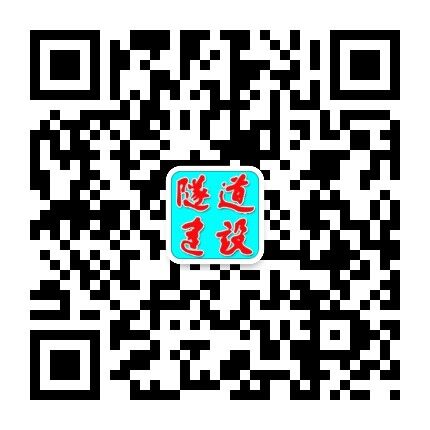
隧道建设(中英文) ›› 2023, Vol. 43 ›› Issue (S1): 550-557.DOI: 10.3973/j.issn.2096-4498.2023.S1.066
孙浩1, 2, 贾连辉1, 魏晓龙1, 林福龙1, 孟祥波1
(1. 中铁工程装备集团有限公司, 河南 郑州 450000; 2.郑州大学机械与动力工程学院, 河南 郑州 450000)
SUN Hao1, 2, JIA Lianhui1, WEI Xiaolong1, LIN Fulong1, MENG Xiangbo1
摘要: 盾构在掘进过程中面临着极其复杂的地质条件,刀具极易损坏,而现有的刀具故障诊断方法无法对刀具故障进行全面、准确的判断。针对现有刀具故障诊断方法的不足,分析SVM、BPNN、ELM、RF等机器学习算法的原理及特点,研究常压刀盘盾构滚刀常见的磨损超限、卡转和偏磨等故障机制及特征,设计基于机器学习的常压刀盘盾构滚刀故障诊断模型,对滚刀故障进行分层诊断。选取对于滚刀故障较为敏感的温度、瞬时转速比、平均转速比等参数,使用盾构掘进现场数据进行试验,用于判断滚刀是否发生故障的第1层SVM、BPNN、ELM和RF算法模型准确率分别达到87.49%、88.69%、78.27%和96.45%,当滚刀发生故障时,用于判断滚刀具体故障形式的第2层SVM、BPNN、ELM和RF算法模型准确率分别达到90.24%、86.76%、79.41%和97.06%。验证了基于机器学习的常压刀盘盾构滚刀故障诊断模型的科学性和有效性,以及RF算法在判断滚刀是否发生故障以及发生故障后滚刀的故障类型具有较高的准确率,能够有效降低企业施工、换刀成本,提高盾构掘进效率。