- 中国科学引文数据库(CSCD)来源期刊
- 中文核心期刊中文科技核心期刊
- Scopus RCCSE中国核心学术期刊
- 美国EBSCO数据库 俄罗斯《文摘杂志》
- 《日本科学技术振兴机构数据库(中国)》
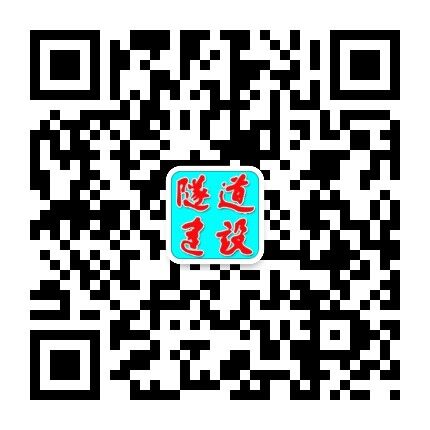
隧道建设(中英文) ›› 2023, Vol. 43 ›› Issue (12): 2066-2076.DOI: 10.3973/j.issn.2096-4498.2023.12.009
孙泽1, 2, 宋战平1, 2, 3 *, 岳波3, 4, 杨子凡2
SUN Ze1, 2, SONG Zhanping1, 2, 3, *, YUE Bo3, 4, YANG Zifan2
摘要: 为充分利用现场监测数据所反馈的围岩变形信息,对岩体力学参数进行反演,以贵州省剑河至黎平高速公路TJ-1标段牛练塘隧道为工程背景,选择围岩弹性模量、黏聚力、泊松比及内摩擦角为影响因素,通过设计正交试验及有限元模拟,获取25组围岩物理力学参数组合及其对应的拱顶沉降值和拱腰收敛模拟值。基于随机梯度下降算法(stochastic gradient descent algorithm,简称SGD算法)对传统BP神经网络模型进行改进,建立以拱顶沉降值和拱腰收敛值为输入参数,以围岩弹性模量、黏聚力、泊松比及内摩擦角为输出值的基于SGD算法优化的BP神经网络模型,实现围岩参数的反演分析。将反演所得的围岩参数代入有限元模型,验证优化BP神经网络模型的可行性和准确性。最后,分析围岩变形及初期支护受力特性并给出施工建议。结果表明: 1)基于SGD算法优化的BP神经网络模型计算得出的拱顶沉降值、拱腰收敛值、拱肩收敛值与现场实测值的相对误差率在2.50%~24.01%,均低于传统BP神经网络模型计算得出的误差率(11.51%~93.71%),验证优化BP神经网络模型的可行性和优越性; 2)上、下台阶拱脚处的喷层和锚杆有应力集中现象,有破坏风险,建议施工中加强拱脚支护,防止发生工程事故。