ISSN 2096-4498
CN 44-1745/U
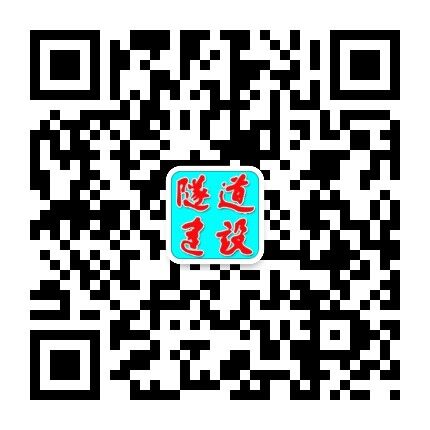
ISSN 2096-4498
CN 44-1745/U
Tunnel Construction ›› 2023, Vol. 43 ›› Issue (7): 1118-1126.DOI: 10.3973/j.issn.2096-4498.2023.07.004
Previous Articles Next Articles
ZHANG Dongliang1, GONG Zhiqun1, YANG Shiting1, LU Yening1, ZHAN Jing2, *, AN Xiaoya3, 4