ISSN 2096-4498
CN 44-1745/U
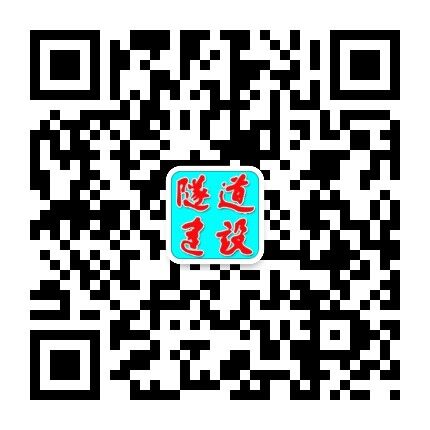
ISSN 2096-4498
CN 44-1745/U
Tunnel Construction ›› 2024, Vol. 44 ›› Issue (4): 673-681.DOI: 10.3973/j.issn.2096-4498.2024.04.005
Previous Articles Next Articles
MAO Qinping1, LIU Juncheng1, YANG Xiaoqiu2, 3, *, LIU Xuezeng4, SANG Yunlong2, 3