ISSN 2096-4498
CN 44-1745/U
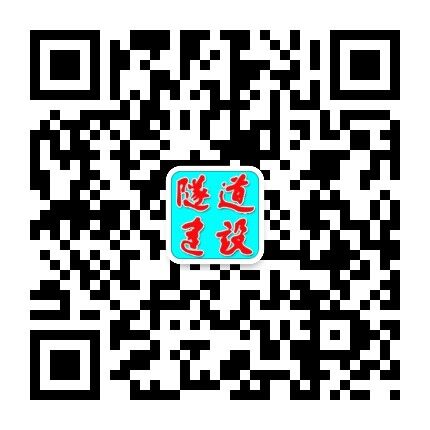
ISSN 2096-4498
CN 44-1745/U
Tunnel Construction ›› 2024, Vol. 44 ›› Issue (6): 1274-1282.DOI: 10.3973/j.issn.2096-4498.2024.06.014
Previous Articles Next Articles
ZHOU Menglin1, CHEN Qiang1, *, WANG Bo2, SONG Ziyuan1, PENG Chuanyang1, CHENG Li1