ISSN 2096-4498
CN 44-1745/U
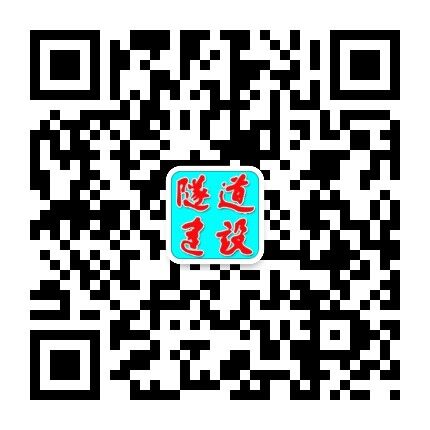
ISSN 2096-4498
CN 44-1745/U
Tunnel Construction ›› 2024, Vol. 44 ›› Issue (10): 1961-1970.DOI: 10.3973/j.issn.2096-4498.2024.10.005
Previous Articles Next Articles
BAO Yan1, MEI Chongbin1, XU Pengyu2, SUN Zhe1, WEN Yucheng1
(1. College of Architecture and Civil Engineering, Beijing University of Technology, Beijing 100124, China; 2. Beijing Urban Construction Exploration & Surveying Design Research Institute Co., Ltd., Beijing 100101, China)