ISSN 2096-4498
CN 44-1745/U
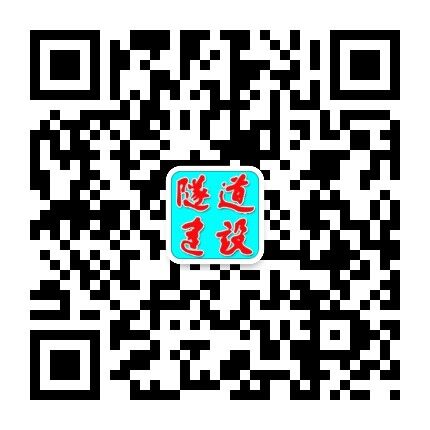
ISSN 2096-4498
CN 44-1745/U
Tunnel Construction ›› 2024, Vol. 44 ›› Issue (10): 2032-2040.DOI: 10.3973/j.issn.2096-4498.2024.10.011
Previous Articles Next Articles
GUAN Zhenchang1, XIE Lifu1, ZHOU Yuxuan1, LUO Song2, XU Chao3