ISSN 2096-4498
CN 44-1745/U
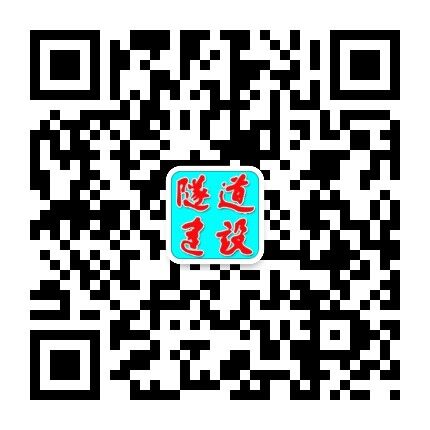
ISSN 2096-4498
CN 44-1745/U
Tunnel Construction ›› 2024, Vol. 44 ›› Issue (11): 2233-2240.DOI: 10.3973/j.issn.2096-4498.2024.11.013
Previous Articles Next Articles
Surface Settlement Prediction Method for Shield Tunneling Based on Stacking Ensemble Learning
ZHENG Yiming1, LI Gang2, JI Jun3, ZHANG Mengxi1, *, WU Huiming2
(1. School of Mechanics and Engineering Science, Shanghai University, Shanghai 200444, China; 2. Shanghai Tunnel Engineering Co., Ltd., Shanghai 200032, China; 3. Shanghai Urban Investment Waterworks Engineering Project Management Co., Ltd., Shanghai 201103, China)