ISSN 2096-4498
CN 44-1745/U
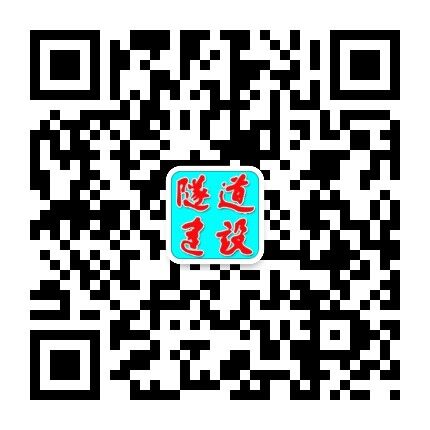
ISSN 2096-4498
CN 44-1745/U
Tunnel Construction ›› 2023, Vol. 43 ›› Issue (12): 1985-1995.DOI: 10.3973/j.issn.2096-4498.2023.12.001
Previous Articles Next Articles
HU Changming1, 2, FENG Lili1, *, LI Liang1, YU Xiangtao3, LI Zengliang3