ISSN 2096-4498
CN 44-1745/U
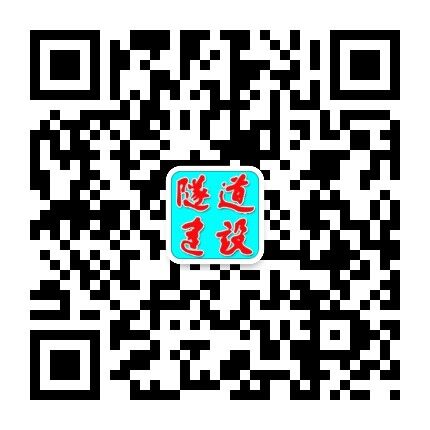
ISSN 2096-4498
CN 44-1745/U
Tunnel Construction ›› 2023, Vol. 43 ›› Issue (12): 2007-2017.DOI: 10.3973/j.issn.2096-4498.2023.12.003
Previous Articles Next Articles
Rock Strength Prediction Model Based on Drilling Parameters of Rock Drilling Jumbo
ZHANG Shucai1, QIU Wenge2, 3, ZHANG Qifang4, ZHANG Yijiang5, JIANG Shuhua3, LUO Jie2, *
(1.Anhui-Jiangxi Railway Anhui Co.,Ltd.,Hefei 230011,Anhui,China;2.Chengdu Tianyou Tunnel Key Company Ltd.,Chengdu 610031,Sichuan,China;3.Key Laboratory of Transportation Tunnel Engineering,the Ministry of Education,Southwest Jiaotong University, Chengdu 610031,Sichuan,China;4.China Railway Shanghai Design Institute Group Corporation Limited,Shanghai 200070,China;5.No.3 Construction Company of China Railway No.10 Engineering Group,Hefei 250101,Anhui,China)