ISSN 2096-4498
CN 44-1745/U
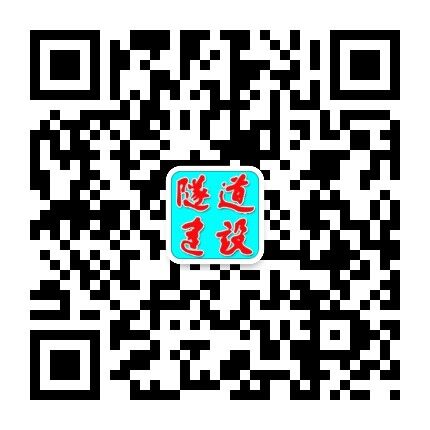
ISSN 2096-4498
CN 44-1745/U
Tunnel Construction ›› 2023, Vol. 43 ›› Issue (12): 2066-2076.DOI: 10.3973/j.issn.2096-4498.2023.12.009
Previous Articles Next Articles
SUN Ze1, 2, SONG Zhanping1, 2, 3, *, YUE Bo3, 4, YANG Zifan2