ISSN 2096-4498
CN 44-1745/U
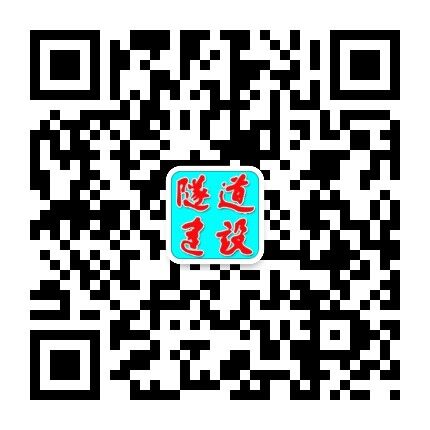
ISSN 2096-4498
CN 44-1745/U
Tunnel Construction ›› 2022, Vol. 42 ›› Issue (S2): 36-43.DOI: 10.3973/j.issn.2096-4498.2022.S2.006
Previous Articles Next Articles
WANG Bozhi1, 2, CHEN Wenming3, 4, XIE Hao1, 2, DING Shuang3, 4, SANG Yunlong3, 4, LIU Xuezeng5, *