- CSCD核心中文核心科技核心
- RCCSE(A+)公路运输高质量期刊T1
- Ei CompendexScopusWJCI
- EBSCOPж(AJ)JST
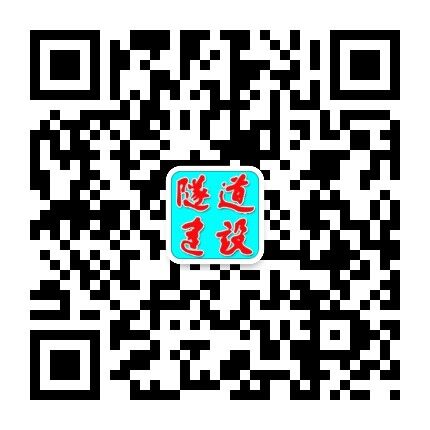
隧道建设(中英文) ›› 2023, Vol. 43 ›› Issue (12): 1996-2006.DOI: 10.3973/j.issn.2096-4498.2023.12.002
赵栓峰, 王帅钧, 李阳, 吴宇尧, 王梦维
SelfCorrecting Point Cloud for Dump Carriage Based on Deep Convolutional SelfCoding Network
ZHAO Shuanfeng, WANG Shuaijun, LI Yang, WU Yuyao, WANG Mengwei
摘要: 为解决智能化盾构施工中,施工隧道内所采集的渣土车原始点云数据存在畸变,从而影响渣土体积测量准确性的问题,基于点云数据处理与深度学习的方法,建立基于深度卷积自编码网络的渣土车厢自适应去畸变模型。首先,采用包围盒滤波和反距离加权插值对渣土车厢点云数据进行滤波与补全操作,接着使用真实渣土车厢尺寸构建网络的理想输出,并通过灰度化将点云数据转换为伪特征图,构建网络的数据集; 然后,以传统卷积自编码网络为基础构建渣土车厢自校正网络,网络设计融合堆叠式卷积层和栈式自编码的处理方法,增加网络层数以获得更优的特征表达; 最后,使用盾构掘进现场数据进行试验。结果表明: 本文提出的渣土车厢点云数据自校正方法在保证时间效率的前提下,渣土车厢数据的峰值信噪比(PSNR)达到29.73,结构相似性(SSIM)达到0.86,均优于传统自编码网络与几何约束矫正的方法。证明了本文方法的正确性与有效性,能够提高隧道内所获取渣土车厢点云数据的可用性,同时为点云数据去畸变技术在三维重构和工程领域的应用提供了理论依据。