- CSCD核心中文核心科技核心
- RCCSE(A+)公路运输高质量期刊T1
- Ei CompendexScopusWJCI
- EBSCOPж(AJ)JST
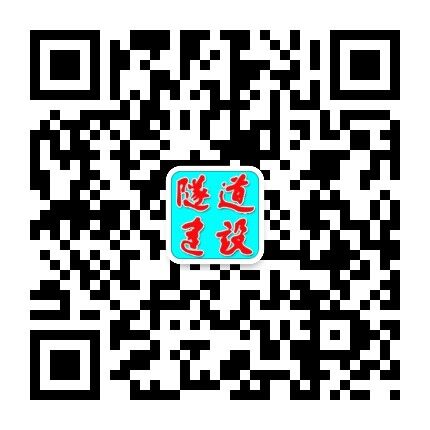
隧道建设(中英文) ›› 2023, Vol. 43 ›› Issue (11): 1853-1861.DOI: 10.3973/j.issn.2096-4498.2023.11.005
顾伟红, 毛梦薇*
Application of Improved Convolution Neural Network in Gearbox Wear Status Recognition of Tunnel Boring Machines
GU Weihong, MAO Mengwei*
摘要: 全断面硬岩隧道掘进机(TBM)变速箱在运行过程中长期承受强转矩作用,导致其磨损故障率较高,为准确识别出变速箱的磨损状态,避免更大故障的发生,提出一种基于改进卷积神经网络(CNN)的TBM变速箱磨损状态识别方法。首先,根据变速箱的磨损状态识别机制,选取光谱分析结果中9种元素的质量分数值作为变速箱磨损状态识别的指标; 然后,建立改进的卷积神经网络模型,使用2层卷积层堆叠代替传统模型中的1层卷积层深度提取数据特征,并使用2个尺寸不同的卷积核提取不同层级的特征; 最后,将该模型应用于实际工程中,将采集到的部分TBM变速箱油液光谱数据和磨损状态标签输入模型进行训练,使用训练好的模型对测试集数据进行磨损状态识别。研究结果表明,该方法能准确识别出TBM变速箱的磨损状态,准确率达到95%,可为实际施工中TBM变速箱的维护保养提供决策支持。