ISSN 2096-4498
CN 44-1745/U
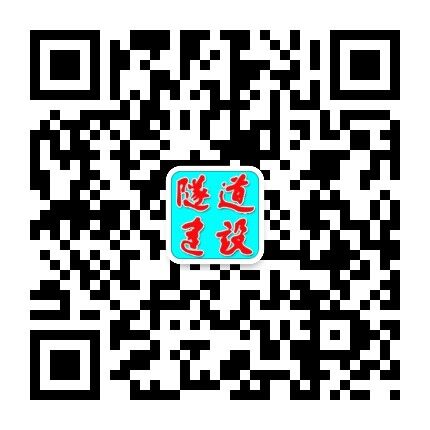
ISSN 2096-4498
CN 44-1745/U
Tunnel Construction ›› 2023, Vol. 43 ›› Issue (S1): 550-557.DOI: 10.3973/j.issn.2096-4498.2023.S1.066
Previous Articles Next Articles
SUN Hao1, 2, JIA Lianhui1, WEI Xiaolong1, LIN Fulong1, MENG Xiangbo1