ISSN 2096-4498
CN 44-1745/U
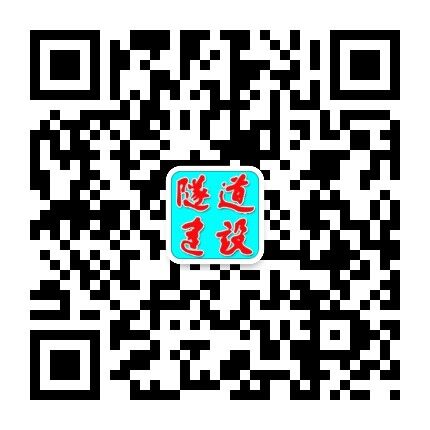
ISSN 2096-4498
CN 44-1745/U
Tunnel Construction ›› 2024, Vol. 44 ›› Issue (11): 2171-2180.DOI: 10.3973/j.issn.2096-4498.2024.11.007
Previous Articles Next Articles
LUO Weibo1, LI Long1, WANG Lai2, *, SUN Jiali1, PAN Qiujing2
(1. CCCC Third Harbor Engineering Co., Ltd., Shanghai 200032, China; 2. School of Civil Engineering, Central South University, Changsha 410075, Hunan, China)