ISSN 2096-4498
CN 44-1745/U
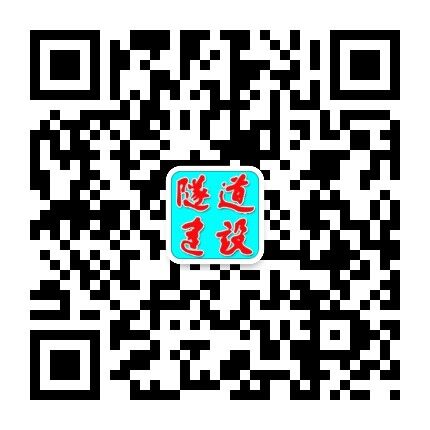
ISSN 2096-4498
CN 44-1745/U
Tunnel Construction ›› 2023, Vol. 43 ›› Issue (11): 1853-1861.DOI: 10.3973/j.issn.2096-4498.2023.11.005
Previous Articles Next Articles
Application of Improved Convolution Neural Network in Gearbox Wear Status Recognition of Tunnel Boring Machines
GU Weihong, MAO Mengwei*