ISSN 2096-4498
CN 44-1745/U
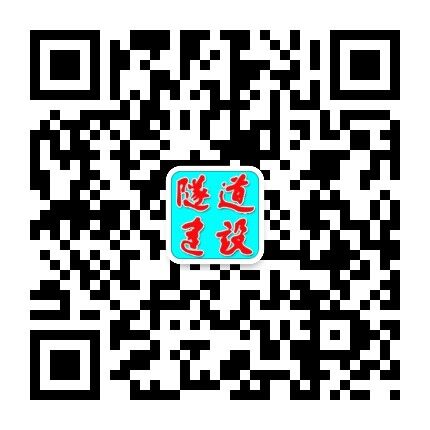
ISSN 2096-4498
CN 44-1745/U
Tunnel Construction ›› 2024, Vol. 44 ›› Issue (7): 1410-1421.DOI: 10.3973/j.issn.2096-4498.2024.07.008
Previous Articles Next Articles
XIA Qinyong1, 2, WANG Mingnian1, 2, *, SUN Hongqiang1, 2, LIN Peng1, 2, ZHAO Siguang3
(1. State Key Laboratory of Intelligent Geotechnics and Tunnelling, Southwest Jiaotong University, Chengdu 610031, Sichuan, China; 2. School of Civil Engineering, Southwest Jiaotong University, Chengdu 610031, Sichuan, China; 3. Railway Engineering Technical Standards Institut, China Railway Economic and Planning Research Institute, Beijing 100038, China)