ISSN 2096-4498
CN 44-1745/U
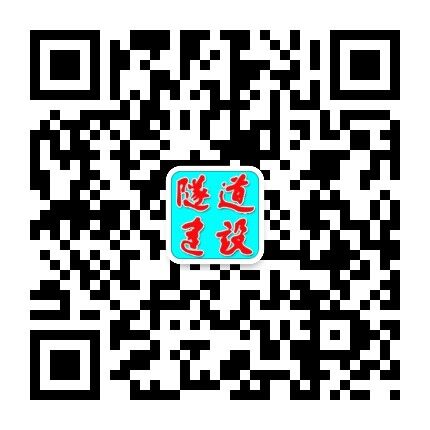
ISSN 2096-4498
CN 44-1745/U
Tunnel Construction ›› 2024, Vol. 44 ›› Issue (5): 1056-1067.DOI: 10.3973/j.issn.2096-4498.2024.05.013
Previous Articles Next Articles
XIANG Lulu1, 2, TONG Jianjun1, 2, *, WANG Mingnian1, 2, MIAO Xingwang1, 2, YE Pei1, 2