- 中国科学引文数据库(CSCD)来源期刊
- 中文核心期刊中文科技核心期刊
- Scopus RCCSE中国核心学术期刊
- 美国EBSCO数据库 俄罗斯《文摘杂志》
- 《日本科学技术振兴机构数据库(中国)》
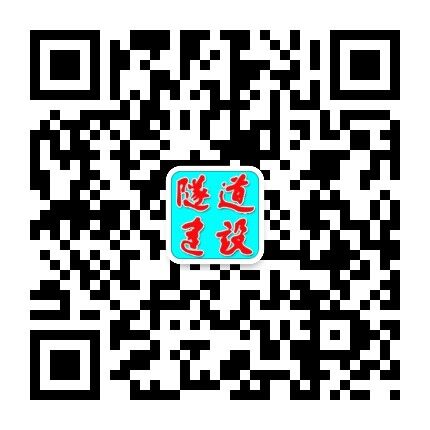
隧道建设(中英文) ›› 2023, Vol. 43 ›› Issue (S1): 304-312.DOI: 10.3973/j.issn.2096-4498.2023.S1.035
刘华吉1, 孙红林1, 张占荣1, 尤明龙2,*,
谭飞2, 李炜1
(1. 中铁第四勘察设计院集团有限公司, 湖北 武汉 430063;2. 中国地质大学(武汉)工程学院, 湖北 武汉 430074)
Intelligent Identification of SandstoneSandy Mudstone Interface Based on Drilling Parameters
LIU Huaji1, SUN Honglin1, ZHANG Zhanrong1, YOU Minglong2, *, TAN Fei2, LI
Wei1
(1. China Railway Siyuan Survey and Design Group Co., Ltd., Wuhan 430063, Hubei, China; 2. Faculty of Engineering, China University of Geosciences, Wuhan 430074, Hubei, China)
摘要: 地层岩体种类的识别是地质和岩土勘察工作的重点内容,为解决地层界面岩性辨识困难的难题,研发了一种随钻测量系统,提出了相应的数据处理及分析方法。采用基于机器学习技术的方法,选取支持向量机(SVM)算法,研究了一种通过随钻参数反演地层岩体种类的方案。得出了如下结论: 建立各参数同孔深数据的对应关系,有利于机器学习数据库的建立和反演方案的实施。随钻监测数据处理原则是: 识别各种非钻进状态及异常状态,建立纯钻进状态的时间—孔深曲线和各随钻参数—孔深变化的曲线。SVM在地层岩体种类识别方面取得了良好的效果,预测结果与钻探记录基本一致,可用于地层关键岩体种类识别,防止虚假钻孔和虚假编录,为岩土工程智能勘测提供了新的途径。