ISSN 2096-4498
CN 44-1745/U
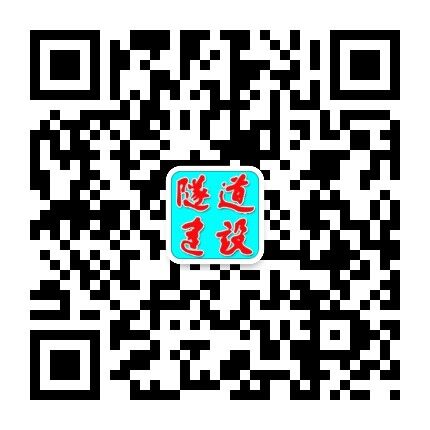
ISSN 2096-4498
CN 44-1745/U
Tunnel Construction ›› 2024, Vol. 44 ›› Issue (3): 484-495.DOI: 10.3973/j.issn.2096-4498.2024.03.006
Previous Articles Next Articles
Identification and Prediction of Composite Ratios for Strata in Metro Shield Tunnel Using Artificial Bee ColonyBack Propagation Neural Network
GUO Yong1, GUO Xiaolin2, JIAN Yongzhou3, ZHANG Jian2, *, FENG Tugen2, CHEN Zi′ang2
(1. Shanghai Huatie Engineering Consulting Co., Ltd., Shanghai 200040, China; 2. Key Laboratory of Geomechanics and Embankment Engineering, the Ministry of Education, Hohai University, Nanjing 210098, Jiangsu, China; 3. CCCCSHB Fourth Engineering Co., Ltd., Luoyang 471013, Henan, China)