- 中国科学引文数据库(CSCD)来源期刊
- 中文核心期刊中文科技核心期刊
- Scopus RCCSE中国核心学术期刊
- 美国EBSCO数据库 俄罗斯《文摘杂志》
- 《日本科学技术振兴机构数据库(中国)》
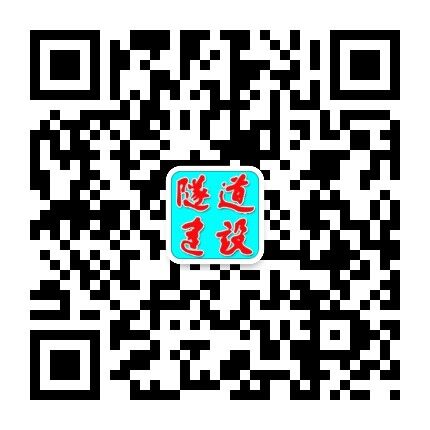
隧道建设(中英文) ›› 2023, Vol. 43 ›› Issue (12): 1985-1995.DOI: 10.3973/j.issn.2096-4498.2023.12.001
胡长明1, 2, 冯丽丽1, *, 李靓1, 于祥涛3, 李增良3
HU Changming1, 2, FENG Lili1, *, LI Liang1, YU Xiangtao3, LI Zengliang3
摘要: 针对盾构施工过程中引发的地表沉降问题,通过优化掘进参数实现对地表沉降的控制,保障施工顺利进行。基于长短期记忆神经网络(LSTM)与粒子群算法(PSO)等方法,提出改进LSTM-PSO掘进参数优化模型。首先,构建长短期记忆神经网络模型预测地表沉降,并与随机森林(RF)和BP神经网络的预测结果进行对比,证明LSTM模型的优越性;然后,采用组合权重改进LSTM模型,对比改进前后的地表沉降预测效果;最后,基于改进LSTM地表沉降预测模型,结合粒子群算法,构建改进LSTM-PSO掘进参数优化模型,将其应用于青岛某地铁盾构工程中并验证其可靠性。研究结果表明: 1)LSTM模型在拟合精度和泛化能力方面均比RF模型和BP模型表现出更加优越的性能;采用组合权重改进LSTM模型,改进后的模型对地表沉降的预测性能得到了进一步提升。 2)采用改进LSTM-PSO模型对掘进参数进行优化后,实际施工中地表沉降监测值均在合理范围内,说明所构建的改进LSTM-PSO掘进参数优化模型具有良好的可靠性和工程实用性。