- CSCD核心中文核心科技核心
- RCCSE(A+)公路运输高质量期刊T1
- Ei CompendexScopusWJCI
- EBSCOPж(AJ)JST
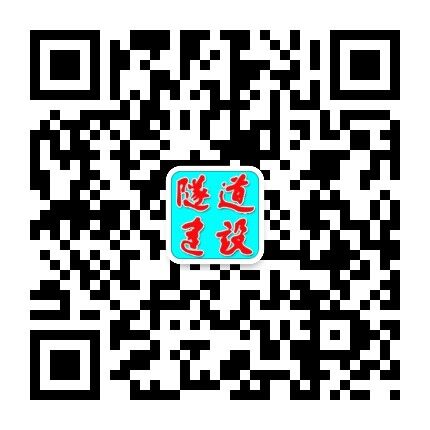
隧道建设(中英文) ›› 2024, Vol. 44 ›› Issue (11): 2119-2132.DOI: 10.3973/j.issn.2096-4498.2024.11.002
杨明辉1, 宋牧原1, 2, *, 姚高占2, 陈伟2, 左国恋2, 蔡智远2
(1. 厦门大学建筑与土木工程学院, 福建 厦门 361005;2. 湖南大学土木工程学院, 湖南 长沙 410082)
Research Progress and Prospects for Machine Learning in Predicting Surface Settlement Induced by Shield Tunneling
YANG Minghui1, SONG Muyuan1, 2, *, YAO Gaozhan2, CHEN Wei2, ZUO Guolian2, CAI Zhiyuan2
(1. School of Architecture and Civil Engineering, Xiamen University, Xiamen 361005, Fujian, China; 2. School of Civil Engineering, Hunan University, Changsha 410082, Hunan, China)
摘要: 针对采用机器学习方法预测盾构掘进地表沉降的研究,围绕预测模型的输入参数、预测目标、算法选取和超参数智能优化4个方面的研究进展开展系统综述,总结出当前研究中亟需解决的关键问题,并展望该领域的未来发展方向。研究表明: 1)结合隧道几何参数、地层参数和盾构操作参数等信息进行沉降预测是当前主流的研究方向; 2)沉降预测前需根据预测目标选取合适的模型和输入参数; 3)通过超参数智能算法优化模型参数以提升预测精度。然而,现阶段的研究仍面临着诸多挑战: 1)预测模型普遍缺乏特征自主识别能力且易发生过拟合; 2)对海量数据的挖掘与分析尚不深入; 3)尚未构建基于多源异构数据集的强鲁棒性模型; 4)对地表沉降发展过程的预测研究相对匮乏。最后,展望盾构隧道智能掘进领域中需重点攻克的难题。