- CSCD核心中文核心科技核心
- RCCSE(A+)公路运输高质量期刊T1
- Ei CompendexScopusWJCI
- EBSCOPж(AJ)JST
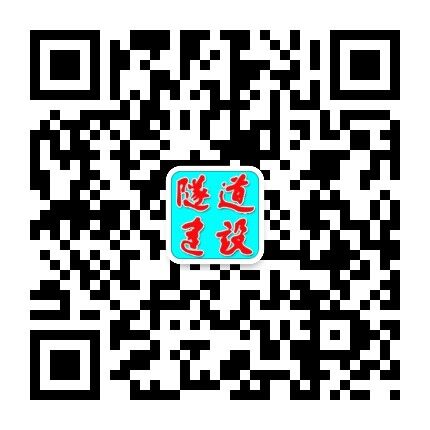
隧道建设(中英文) ›› 2023, Vol. 43 ›› Issue (7): 1118-1126.DOI: 10.3973/j.issn.2096-4498.2023.07.004
张栋樑1, 宫志群1, 杨世廷1, 陆业宁1, 占静2, *, 安晓亚3, 4
ZHANG Dongliang1, GONG Zhiqun1, YANG Shiting1, LU Yening1, ZHAN Jing2, *, AN Xiaoya3, 4
摘要:
针对当前隧道影像拼接过程中效率低、累积误差大的问题,研究提出一种联合SIFT-GPU(scale-invariant feature transform-graphics processing unit)协同处理算法和整体平差法的隧道影像拼接技术。首先,基于车载移动测量技术获取隧道内部的影像和点云数据,利用点云数据和共线方程对原始影像进行纠正处理,并先后使用MASK匀光算法和Wallis滤波匀光法对影像进行匀光匀色; 然后,融合SIFT匹配算法和GPU-CPU(central processing unit)协同处理技术加速提取所有待拼接影像特征点,使用RANSAC(random sample consensus)算法剔除误配点;最后,使用平移旋转缩放变换模型对400张影像进行拼接,采用整体平差算法对拼接结果进行误差补偿,利用GDAL(geospatial data abstraction library)分块处理技术输出拼接成果。研究结果表明: 1)相较于原始的SIFT匹配算法,研究使用的SIFT-GPU协同处理算法使影像匹配效率提高了3~25倍; 2)整体平差算法较好地解决了随着待拼接影像的增加而引起的误差累积问题,相较于传统算法,误差降低至原来的1/3。