- CSCD核心中文核心科技核心
- RCCSE(A+)公路运输高质量期刊T1
- Ei CompendexScopusWJCI
- EBSCOPж(AJ)JST
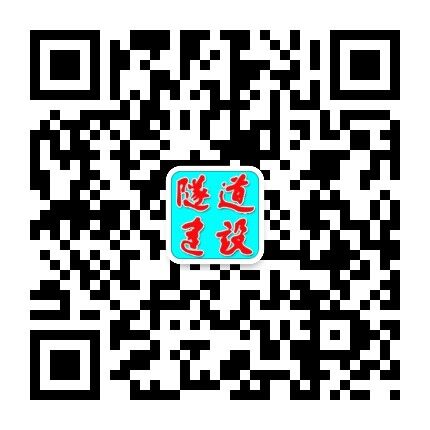
隧道建设(中英文) ›› 2024, Vol. 44 ›› Issue (3): 484-495.DOI: 10.3973/j.issn.2096-4498.2024.03.006
郭勇1, 郭小霖2, 简永洲3, 张箭2, *, 丰土根2, 陈子昂2
(1. 上海华铁工程咨询有限公司, 上海200040; 2. 河海大学 岩土力学与堤坝工程教育部重点实验室,江苏 南京210098; 3. 中交二公局第四工程有限公司, 河南 洛阳471013)
Identification and Prediction of Composite Ratios for Strata in Metro Shield Tunnel Using Artificial Bee ColonyBack Propagation Neural Network
GUO Yong1, GUO Xiaolin2, JIAN Yongzhou3, ZHANG Jian2, *, FENG Tugen2, CHEN Zi′ang2
(1. Shanghai Huatie Engineering Consulting Co., Ltd., Shanghai 200040, China; 2. Key Laboratory of Geomechanics and Embankment Engineering, the Ministry of Education, Hohai University, Nanjing 210098, Jiangsu, China; 3. CCCCSHB Fourth Engineering Co., Ltd., Luoyang 471013, Henan, China)
摘要: 为研究盾构掘进过程中掘进参数与地层情况的关联性,建立盾构掘进过程中的机-岩关系,依托南京地铁6号线某盾构施工区间数据进行复合地层下掘进参数的统计分析。首先,利用掘进参数与地层的相关性,采用人工蜂群算法优化的BP神经网络,建立可根据掘进参数识别开挖面地层并描述复合地层组合情况的ABC-BP神经网络模型; 然后,针对盾构区间进行地层识别和区间内2种复合地层的复合比预测。结果表明: 1)盾构掘进参数的波动范围与均值随开挖面所处地层变化,且依地层不同呈现一定规律性; 2)地层类别预测结果表明,模型对上软下硬地层、中风化泥质砂岩、粉质黏土的识别召回率分别为94.1%、96.6%、96%,总体识别准确率为95%; 3)针对复合比的预测结果表明,相较于其他机器学习模型,ABC-BP模型的平均绝对误差、均方根误差均减小且样本回归值提升,在预测精度和预测稳定性方面具有一定的优越性。
中图分类号: