ISSN 2096-4498
CN 44-1745/U
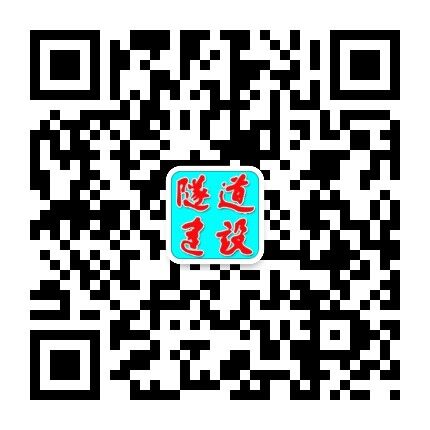
ISSN 2096-4498
CN 44-1745/U
Tunnel Construction ›› 2024, Vol. 44 ›› Issue (11): 2139-2148.DOI: 10.3973/j.issn.2096-4498.2024.11.004
Previous Articles Next Articles
ZENG Yi1, WU Jiamin1, BIAN Yuewei1, TANG Jiayou2, YAN Tao2, SHEN Shuilong2, *